In this thesis, we propose a trainable filter which recovers most of chest data modified by chest tube attenuations. It describes how you may remove the chest tube figure (as an example of artificial object might be presented at the radiography) from Postero-Anterior (PA) Chest Radiography.
We decide to focus on this issue, because the chest radiography is the most common radiological modality in the practice. Especially, it is very popular for scanning and screening purposes. Unfortunately, at the same time it is one of the most difficult radiological modalities. The overlapping tissues cause a highly complex projection. In addition, artificial objects such as catheters, chest tube, pacemaker, and/or even cloths might be presented at this projection image. It is obvious that the anomaly detection algorithm should not be confused by these objects.
To achieve this goal, we study to train a Convolutional Neural Network (CNN) that gives an artificial x-ray without a chest tube as output for a given x-ray image which contains the chest tube as an input.
The results show that our model can remove chest tube figures from radiographies. We believed that our model gives promising results as a starting point for an artificial object removing problem. We show that a module to protect the Computer Aided Diagnosis (CAD) system’s accuracy from errors caused by foreign objects is possible.
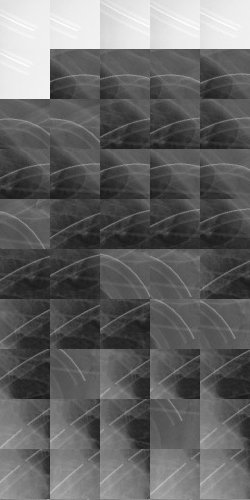
Click here for next page >>